-
Written by Sean Dougherty
A copywriter at Funnel, Sean has more than 15 years of experience working in branding and advertising (both agency and client side). He's also a professional voice actor.
Today, measurement confidence is low among marketers. While attribution modeling helps teams understand which efforts drive conversions — used in 36% of campaigns — two-thirds still struggle with maintaining a consistent measurement framework.
Attribution models alone have left marketers in a bit of a measurement mess because the first- and third-party data that powers them is getting scarce. As a result, marketers are stuck using data they don’t find meaningful, describing 39% as “digital vanity metrics” in 2023.
Figuring out how to fix this mess can only happen by understanding how we got here.
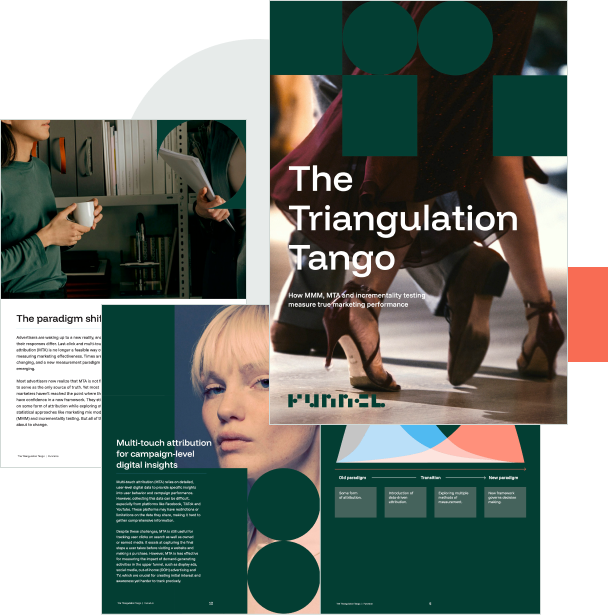
Still relying solely on attribution to gauge your marketing success? Discover how to integrate MMM, MTA, and incrementality testing for a more precise and comprehensive performance measurement in this insightful eBook.
Schedule your free demo
What is marketing measurement?
Marketing measurement is the process of collecting and analyzing data from online and offline channels, like digital advertising, out-of-home (OOH), email marketing, events and your website. It can cover anything from clicks on an ad to form fills on a website, but common examples like these don’t prove your marketing efforts contributed to your company’s financial gain.
However, some metrics do connect efforts to sales — think marketing’s impact on sales, customer lifetime value (CLV), customer acquisition cost (CAC) and leads. The problem is that gathering these marketing metrics has historically been hard.
A good measurement framework should boost your confidence in your marketing strategy. It should help you decide whether your money is in the right place and if you’re spending time on high-value marketing efforts.
The cycle of marketing measurement.
Marketers have struggled to measure key performance indicators (KPIs) that connect effort to sales in part because of the way measurement has evolved. We used to be able to track user behavior and attribute conversions through cookies and pixels with ease. Today, customers want more privacy and for businesses to collect less of their data. As a result, the attribution models of the past are suffering.
But there’s a new measurement philosophy that compensates for attribution’s flaws. It combines the strengths of several measurement methods to minimize the limitations of each. This new method, called triangulation, combines today’s familiar attribution models with probabilistic ones using increasingly abundant and reliable data.
The evolution of marketing measurement
Marketing measurement has come a long way since 1923 when Claude C. Hopkins first suggested it could be a science. Marketing teams didn’t really buy into the science idea until digital ads took off. And when they did, it started a long-winded pursuit of attribution models that marketers could stuff with data from ads, social media, website traffic and customer relationship management (CRM) systems.
You’d think all this data would make it easy to determine which marketing campaigns are actually working, but the opposite is true. New privacy regulations and restrictions from platforms like Meta, Google and TikTok make it hard to get a clear picture. The regulations restrict marketers from collecting data from third parties, while the platforms claim full credit for conversions, whether other factors played a role or not.
This doesn’t just mess up reporting. It makes it hard to get brand and performance marketing on the same page. Rather, they run in silos with different business goals and marketing metrics.
The technology behind cookies and pixels is at the heart of all this confusion.
How data collection has evolved
Early online ads were pretty simple to measure — you’d count clicks and impressions, kind of like how TV and radio measure views or listeners. But then tracking pixels and cookies came along and changed everything.
Suddenly, marketers could link ad views directly to conversions, and it got a whole lot easier to get granular with marketing ROI. That kicked off the pursuit of “perfect attribution,” where marketers tried to map every single step a person took before a purchase.
Think of a cookie like a sticky note. When you visit a site, it’s like that site leaves a sticky note on your browser. The note has reminders, like login details, items in your shopping cart or language preferences.
First-party cookies can only be read by the sites that left them. Third-party cookies are notes from other sites that want to help advertisers see where you’ve been. Then, tracking pixels use those cookies to track when you see or click an ad.
First-party vs. third-party cookies.
As helpful as these were for marketers, people got fed up with feeling tracked everywhere they went online. Privacy concerns became so big that Google even announced they’d stop using third-party cookies altogether, then pulled back when they realized how tricky it would be. Still, the phase-out is on its way, and new privacy laws are making it harder for marketers to collect data as freely as they used to.
How privacy regulations impact data collection
New privacy regulations, like Europe’s GDPR introduced in 2018, have dramatically changed the landscape for marketing measurement. They require companies to get consent before tracking consumers with cookies. When consumers opt out, tracking becomes less consistent.
Apple’s Intelligent Tracking Prevention (ITP), introduced in 2017, restricts cookies on Safari to just a few days. Plus, ad blockers, which block scripts that track consumers across visits, are becoming more popular. This makes it hard for tools like Google Analytics (GA) to track users over time.
Privacy regulations and industry influences on measurement.
As these regulations and tracking restrictions increase, marketers realize that attribution models are never going to be perfect. Attribution often gives too much credit to specific marketing channels. Also, you might have multiple platforms claiming credit for the same conversion, leading to double counting.
How data has been analyzed
Some still rely on attribution to measure marketing performance and prove their impact. For years, they believed enough data on their target audience could paint a clear picture of every touchpoint.
If someone clicked on an ad before making a purchase, an attribution model would give that ad some credit for the sale. But, these models started to show their limits when people clicked on multiple ads before buying. Then, the total credit given often added up to more conversions than the number that actually occurred.
To solve this, marketers started using tools like Google Analytics to split credit among different ads and channels. GA allows marketers to benefit from various attribution models, starting with simple ones like last-touch and first-touch, which give all the credit to either the last or first ad clicked. These are still the easiest to use but tend to focus on short-term results rather than building a brand over time. More detailed models called multi-touch attribution (MTA) or heuristic models, spread the credit across multiple ads, giving a fuller picture of the customer journey.
In 2013, Google introduced data-driven attribution, which uses machine learning (ML) to figure out which ads are most likely to lead to sales. This provides a closer look at how individual ads perform but mainly focuses on Google Ads. Facebook and other platforms created their own versions, which can be unreliable due to each platform’s incentive to favor its own channel.
Attribution models from least advanced to most advanced.
Ultimately, this relatively modern attribution approach is falling apart as privacy rules become stricter and data becomes harder to collect. Google Analytics relies on HTTP referrer data, cookies and UTM codes to link marketing campaigns to sales, but these data sources are becoming harder to access.
The future: probabilistic marketing measurement
The attribution approach to marketing measurement quantifies deterministic data — exact information collected directly from consumers through things like cookies. On the other hand, probabilistic data makes educated guesses about a consumer’s identity using signals like device type and location. Though less precise, it fills the gaps left by the decline of cookies.
Probabilistic data also supports methods like marketing mix modeling (MMM) and incrementality testing, which help marketers shift their focus back to long-term brand-building rather than short-term sales. AI and machine learning (ML) play a big role here. They’re the reason marketers can produce and process probabilistic data in real time and identify patterns that would otherwise be impossible to track.
Marketing mix modeling
Marketing mix modeling (MMM) is a method from the 1960s that uses non-linear statistical regression. That’s a fancy way to say you’re fitting a curve to scattered data points on a graph — it reveals patterns a straight line wouldn’t.
It does this by calculating how things like ad spending, seasonality and competitor actions have influenced sales in the past and estimates how adjusting the marketing mix could boost sales.
There are two main ways to approach it: frequentist and Bayesian statistics.
Statistical models used in marketing mix modeling.
Frequentist statistics only use the data in front of you without factoring in any prior knowledge. This keeps things unbiased but can sometimes lead to odd conclusions. For example, your model might show Google Ads hurt sales in March, but in reality, this was due to a dip in demand after the holidays rather than the ads themselves.
Bayesian statistics starts with a hypothesis, like “Google Ads usually have a seasonality dip in March.” Then, it updates that belief with new data. This can prevent unrealistic results, like suggesting that Google Ads aren’t effective when they are, but it introduces some bias.
Marketing mix modeling can be tailored for your company. You can start by defining specific questions and gathering a full set of relevant historical data. Be sure to plan for a three to six-month setup. Once you’re up and running, MMM is great for understanding how different channels or campaigns are performing, but it isn’t really built for tracking individual customer journeys or super detailed insights at the ad level.
Incrementality testing
Incrementality is a measurement framework for gaining insights at the channel level. Its goal is to determine whether a specific advertising channel truly increases sales.
It does so by comparing the results of a group exposed to ads, called a treatment group, to one that wasn’t, called a control group. To set this up, you need to:
- Have a good sample size: Choose a random, well-sized sample. Larger sizes boost accuracy.
- Decide what to analyze: Measure individual behavior, regions or time periods.
- Focus on statistical significance: The sample size and evaluation method influence confidence.
- Repeat the study: Conditions change, so regular testing keeps results relevant.
- Use common sense: If data looks off, something likely went wrong.
Remember, statistics are a guide, not a replacement for expertise. People can move, and ads might not get served in some locations. Gut-check your results with your experience.
These studies are complex and need a blend of careful planning, statistical skills and marketing insight to get the best answers.
Multi-touch attribution (MTA) still rules today
Multi-touch attribution isn’t going anywhere. It’s still a good way to measure marketing results at the campaign and ad levels, and it will be part of marketing’s new measurement paradigm.
Multi-touch attribution focuses on tracking many touchpoints across a customer journey, and digital marketers get a detailed look at how each ad or campaign contributes to conversions — that’s valuable for optimizing conversions in real time. It’s also important to guide day-to-day budget shifts and respond quickly to changes in consumer behavior.
But, digital privacy rules are making third-party tracking less reliable, limiting the effectiveness of using MTA alone. Advertisers are accepting this truth at different rates.
Those sticking with MTA as a standalone marketing measurement strategy will struggle to get a clear picture of the long-term impact or the full value of offline and cross-channel marketing.
Some are still fully committed to MTA, while others are experimenting with other methods to address its gaps. If we imagine a scale from traditional MTA to a new, yet-to-be-defined standard, the progression might look something like this:
The evolution of marketing measurement toward a new paradigm.
Multi-touch attribution is still a good way to understand which online ads drive conversions if you focus mostly on digital channels. But as your goals get more complex, you should find yourself relying on MTA for tactical decisions and moving toward triangulation to fill in the gaps.
Embrace the new era of marketing measurement
One way to compensate for MTA’s limitations is to supplement it with other measurement methods. Ideally, your measurement strategy consists of daily optimizations and big-picture insights to inform your marketing activities. This is the power of a layered approach: you’re not relying on just one method but can tap into a mix that reveals the full picture.
Marketing triangulation: a new measurement framework.
This layered approach is triangulation — marketers get the value of three different measurement tools that compensate for each other’s limitations.
Marketing measurement is changing fast, and a more holistic approach helps you get past limited data to see the big picture across all your channels. By blending MTA, MMM and incrementality, marketing triangulation gives you a clearer view of what’s working.
Check out the Triangulation Tango to learn how to build your own model.
-
Written by Sean Dougherty
A copywriter at Funnel, Sean has more than 15 years of experience working in branding and advertising (both agency and client side). He's also a professional voice actor.