Contributors
-
Written by Christopher Van Mossevelde
Head of Content at Funnel, Chris has 20+ years of experience in marketing and communications.
-
Reviewed by Janos Moldvay
CEO & Founder of Adtriba
-
Reviewed by Tim Kreienkamp
Tim Kreienkamp is the Chief Data Scientist at Adtriba. He knows all about attribution, data science, marketing analytics and MMM.
%20(2).png)
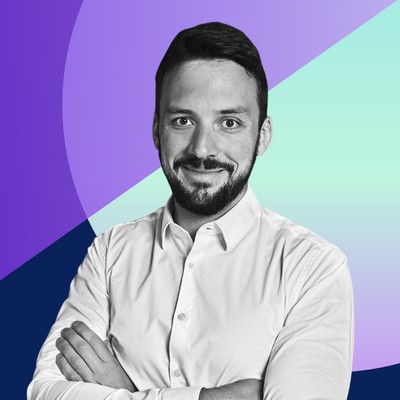
Marketing mix modeling (MMM) and multi-touch attribution (MTA) are key methods in marketing analytics. While MMM offers a macro-level view of marketing impact on revenue, MTA provides granular insights into the effectiveness of specific marketing channels. Understanding when and how to use these methods is crucial for optimizing marketing strategies and maximizing ROI.
While 59% of marketers consider themselves highly data-driven, a significant 41% struggle with data collection, analysis, and reporting. This skills gap hinders the ability to identify the most effective channel combinations for conversion and return. This is where MMM and MTA come into play, helping marketers leverage their data to make informed decisions.
Both MMM and MTA offer valuable insights into marketing effectiveness. Understanding these models is instrumental for modern marketers. However, to fully exploit the benefits of these models, it's essential to understand their distinct functions and appropriate applications. This guide aims to thoroughly explore these aspects.
Measuring marketing effectiveness
Marketing effectiveness is crucial to any business — but how do you measure success, and ensure you are on the right track? You do so by leveraging critical metrics and insights.
While many marketing analytics tools can provide a basic indication of what's working, data is often missing, disconnected, or just misunderstood. That is why you need to understand marketing effectiveness and how it's measured.
Marketing effectiveness determines how well a company's marketing strategies increase revenue while decreasing costs. As a marketer, it helps you determine the true impact of your marketing efforts. After all, that's your primary job — to help increase sales at a lower investment.
Also read: What is MER (marketing efficiency ratio)?
To boost marketing effectiveness, you will determine what campaigns drive value and performance. As you collect data and analyze it, you will be able to make changes that increase company revenue while decreasing customer acquisition costs. The best way is to link sales to various marketing touch points and historical data points.
Some of the benefits of measuring marketing effectiveness include:
- Gaining a better understanding of which leads are most likely to convert and which marketing campaigns deliver the highest quality leads.
- Knowing how to optimize your budgets across varying platforms to achieve the greatest possible ROI.
- Learning how to optimize your output to increase the bottom line.
Marketers are always looking for ways to improve performance. If you can relate, you must start tracking critical marketing effectiveness metrics. These include return on investment (ROI), customer acquisition cost, cost per lead, customer lifetime value, click-through rates, and engagement levels.
Read more: 10 essential performance marketing metrics to monitor
What is multi-touch attribution?
Multi-touch attribution (MTA) is a marketing measurement method that examines all touch points within the customer journey. The goal is to measure the effectiveness of each channel or touchpoint so marketers know where to focus their efforts and resources. By seeing how much each touchpoint contributes to a sale, they can dissect the customer journey to allocate their budget more effectively.
By assigning values to various customer journey touch points, you can more easily determine which marketing channels weigh most heavily in the conversion process. These values are typically applied as percentages.
For example, say someone is seeking a new skincare product. During their research, they may see a display ad from a beauty company. They ignore it but later see a native Facebook ad, bringing them to the company's site. They sign up for the email newsletter, hoping to get a good deal. A week later, they receive a promotional offer via email, and they make the purchase. Each of these marketing initiatives represents a different touchpoint:
- Display ad
- Ad on Facebook
- Email newsletter
When leveraging multi-touch attribution, the marketing team could look at these touch points to see that the native ad and email campaign were effective while the display ad was not. So, maybe they get 35% (Facebook ad), 55% (email), and 10% (display ad) credit. If this trend continues, they will shift away from display ads to pour those resources into a more effective touchpoint.
There are several models within multi-touch attribution, including the following:
- Linear attribution, where equal credit is given to every touchpoint, assuming they are all equal.
- Time-decay attribution gives more credit to touch points near the end of the customer journey, assuming that each touchpoint more strongly influences purchasing behavior.
- Data-driven attribution uses machine learning to analyze how each touchpoint in the customer journey contributes to conversions. It attempts to assigns credit to touchpoints based on their actual impact.
Other attribution models that are commonly used are not multi-touch, but single-touch. They just use one single touchpoint and give credit to that channel. For example:
- First-touch attribution, where conversion credit is given to the first touchpoint — such as a Google search.
- Last-touch attribution, where conversion credit is given to the last touchpoint. It is similar to last-click attribution (which is when full credit is given to the last link a customer clicked on before making a purchase).
The pros of MTA
The advantages of MTA include:
- You can make more informed, data-driven decisions.
- The ability to embrace greater segmentation and personalization.
- Understand sequence of customer journeys on a granular/campaign level
- While data-driven MTA usually includes many thousands of data points (all clicks per visitor), the data necessary to start MTA can be collected in weeks, as opposed to years for MMM.
The cons of MTA
The cons of MTA include:
- The methodology focuses on the campaign level instead of the business level (like with MMM).
- MTA doesn’t account for non-marketing and external effects (baseline, seasonality, product changes) and thus it’s harder to understand incrementality
- Doesn’t include non-trackable channels, such as OOH or TikTok ad views
- It can take a while to implement, especially if you want to take into account many different channels and touch points because MTA relies heavily on integrating data from various channels and platforms.
- With increasing data privacy regulations (like GDPR and CCPA), capturing and utilizing user-level data (which MTA requires) can be problematic.
Marketing mix modeling
Marketing mix modeling (MMM) is an approach that focuses on analyzing sales and historical marketing data to determine which activities have the most significant impact on revenue — or whatever variable you're measuring. Much like multi-touch attribution, the goal is to maximize your marketing budget to distribute resources to the right campaigns and channels.
With MMM, you'll consider the combined impact of varying elements, ranging from advertising spending and promotions to pricing and distribution channels. By examining this historical data, you can identify the relationship between sales and these elements.
The variables you can monitor are nearly limitless but can be grouped into several categories, such as:
- Media activities/marketing tactics, focusing on all of your advertising methods
- External variables include macroeconomic factors, natural disasters, competitor's activities, etc.
- Internal variables include product distribution, product changes, price changes, etc.
- Calendar-based variables, like seasonal trends
This approach is completed based on a series of steps, which include:
- Initial data collection of marketing variables like sales information, expenditures, distribution channels, pricing, etc.
- The collected data is analyzed statistically to determine relationships or patterns.
- Based on the results of this analysis, you would build a customized MMM model that represents marketing actions and sales results. Here, you'll account for external factors and the impact of seasonality changes.
- You can then run what-if simulations to understand how adjustments will influence business results. The goal here is to optimize your strategy and enhance decision-making. Based on your findings, you'll adjust budgets and strategies.
- MMM models are continuous and require ongoing monitoring and updating.
The pros of MMM
MMM provides the following advantages:
- You will gain a holistic view and understand incrementality and marginal CPA or ROAS.
- Offers data-driven insights based on target KPIs and your brand's context/environment.
- Allows for optimal resource allocation.
The cons of MMM
The disadvantages of MMM include:
- The need for large volumes of data that are complete and accurate — for some, that's challenging.
- The need for advanced tools and expertise.
- Data collection, analysis, and modeling can be a high-effort process.
The differences between MMM and MTA
While both methods can provide invaluable insights, they differ. Marketing mix modeling uses statistical techniques to analyze inputs (e.g., channels and budgets) and how they influence outputs (e.g., brand awareness and revenue). In contrast, multi-touch attribution uses a chain of trackable customer interactions to understand the importance of each touchpoint.
So, the primary difference is the objective of each approach. MTA focuses on the impact of specific, individual touch points and how they influence sales/conversions. Using this approach, you can see which touch points were responsible for a sale or conversion. In contrast, MMM focuses on the overall impact of your marketing mix and how that combination influences sales or other outcomes.
Another key difference is the type of data each approach uses. MTA utilizes granular data at the device level, compared to MMM, which uses aggregated data at the channel or campaign level.
These varying approaches mean each has pros and cons based on different use cases.
When to use each model?
The best model will depend on several variables, as both MMM and MTA have strengths and limitations. In some cases, one will be a better option — and in other cases, you may want to lean on both.
Consider these factors:
- Your business objectives
- The quality and availability of your data
- Time sensitivity
- The expertise and resources you'll need
- Cost and budget considerations
When to use MMM
The strongest advantage of MMM is that it's designed for a privacy-first era. If you're well-established and have years of data, MMM can yield meaningful, high-level analysis opportunities.
Privacy restrictions change existing paradigms in what seems like an instant, which is where MMM shines. Increasing privacy regulations is something that's on every marketer’s mind in response to the decline of third-party cookies. Big players like Apple and Google are also changing the game, as they strip UTM parameters and implement other user privacy changes.
Since MMM doesn't capture individual interactions, you don't need to track users with event-tracking solutions — making this a privacy-friendly strategy. Unlike MTA, which focuses on individual touch points, MMM analyzes aggregate historical data from channels and sales. So, it's immune to the changing privacy landscape.
To produce more credible results, combine MMM with tests and experiments (like incrementality measurement). This approach will enhance and validate MMM tactics.
When to use MTA
In the case of MTA, its advantage is that it is more granular than MMM. So, while this isn't great for privacy concerns, it does help you to understand the impact of individual touch points to optimize and improve the effectiveness of campaigns.
Use MTA when you require tactical insights, focusing on short-term optimization.
When to use both
MTA and MMM are powerful tools when used correctly. They can also achieve powerful results when implemented separately. However, knowing when to use both can be a transformative experience. Combining the methodologies results in a unified measurement approach, as discussed below.
MMM offers a top-down, macro-level view of your marketing strategies and efforts, whereas MTA yields a bottom-up, granular view.
What is unified marketing measurement (UMM)?
Unified marketing measurement (UMM) is a method that combines aggregate data and insights from different models, including MMM and MTA, into a more holistic measurement. This framework creates a single source of truth so that you can make real-time adjustments to your campaigns. Think of this approach as the integration of MMM and MTA.
UMM allows to make the impact non-deterministic trackable marketing activities (Tv, social & display ad views, OOH, print) measureable on a customer journey level. This is also the answer on how to measure display ad views after the deprecation of the 3rd party cookie next year.
How to include results from experiments and incrementality tests
Changes within the digital ads ecosystem are helping MMM make a comeback.
For many years, marketers were interested in precise, immediate, and deterministic attribution. However, as discussed, new privacy concerns are making this approach more challenging.
Using MMM methods, you'll want to leverage experimental calibration. Calibration in this context refers to the process of fine-tuning and validating the MMM using experimental data. A case study from Harvard Business Review shows that using calibration with MMM studies can make the models up to 15% more accurate.
This improvement in accuracy means MMM, when calibrated with experimental data, aligns more closely with the actual effectiveness of marketing campaigns, leading to better decision-making.Based on the advantages of MMM and experimental calibration, this approach may become a new gold standard for ad measurement.
To calibrate your MMM, consider the following:
- Compare MMM and ad experiment results to ensure similarity — for example, they should both directionally agree. If they do not, tweak the MMM. This qualitative approach is relatively easy to implement.
- For a more rigorous approach, leverage experiment results to select the best models. Build varying models and then pick those that most closely agree with the ad experiment results based on your outcome of interest.
- If you have a deeper understanding of statistical modeling, you can incorporate experiment results into the MMM.
Note: Be aware that ad experiments and MMM can vary in scope when calibrating. For example, all your advertising efforts (online and offline) vs. digital only. So, there can be interaction effects between online and offline ads and your sales — and vice versa.
Suppose you have the budget and means to utilize MMM. In that case, it is an excellent solution moving forward — and as AI and computing advance, MMM will likely become more efficient and affordable.
Disclaimer: The featured image for this article was created using generative AI.
Contributors
-
Written by Christopher Van Mossevelde
Head of Content at Funnel, Chris has 20+ years of experience in marketing and communications.
-
Reviewed by Janos Moldvay
CEO & Founder of Adtriba
-
Reviewed by Tim Kreienkamp
Tim Kreienkamp is the Chief Data Scientist at Adtriba. He knows all about attribution, data science, marketing analytics and MMM.
%20(2).png)
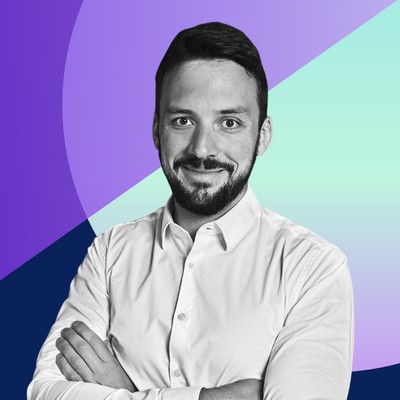